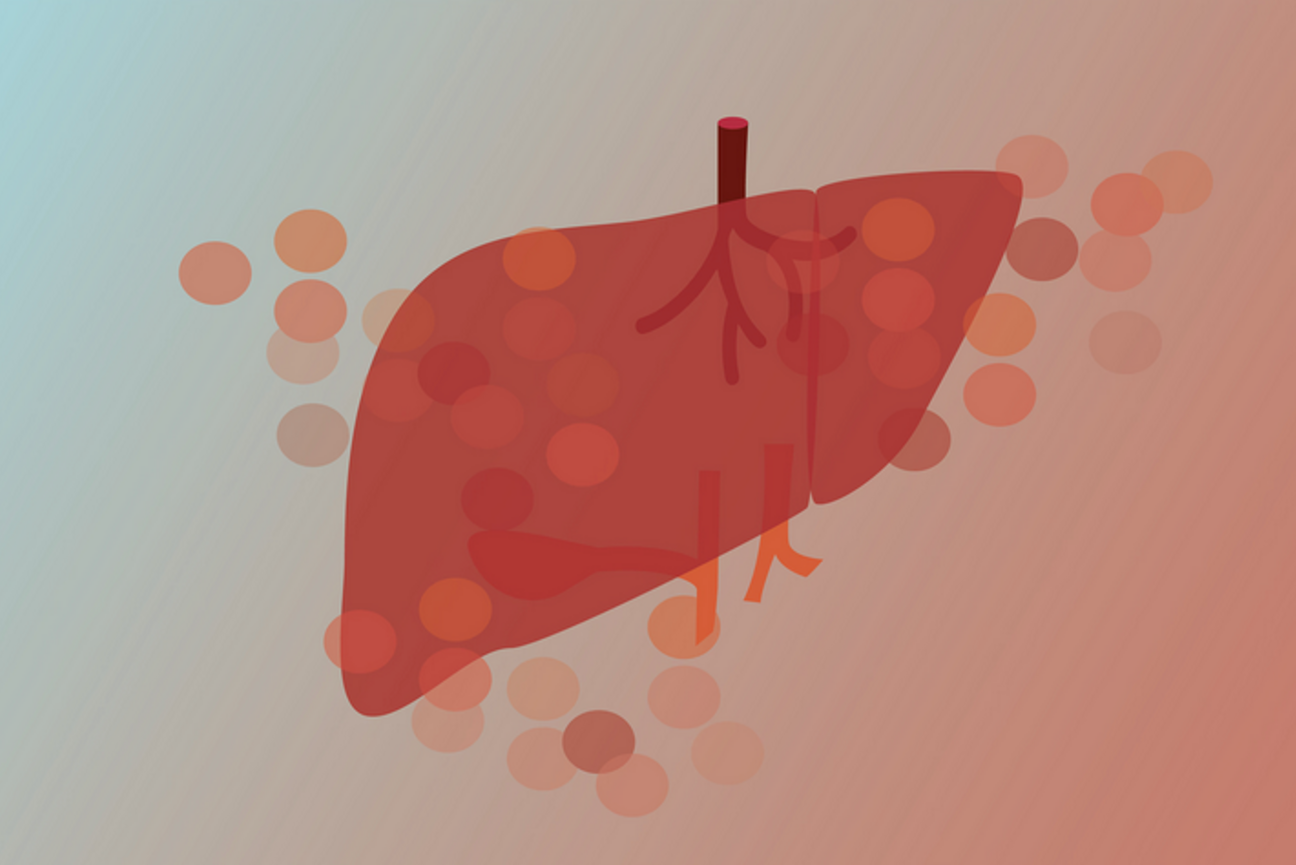
Researchers in the Weissman Lab have developed a powerful approach that simultaneously measures how genetic changes such as turning off individual genes affect both gene expression and cell structure in intact liver tissue, with the goal of discovering how genes control organ function and disease.
Whitehead Institute
June 12, 2025
However, capturing both the “visuals and sound” of biological data, such as gene expression and cell structure data, from the same cells requires researchers to develop new approaches. They also have to make sure that the data they capture accurately reflects what happens in living organisms, including how cells interact with each other and their environments.
Whitehead Institute and Harvard University researchers have taken on these challenges and developed Perturb-Multimodal (Perturb-Multi), a powerful new approach that simultaneously measures how genetic changes such as turning off individual genes affect both gene expression and cell structure in intact liver tissue. The method, described in Cell on June 12, aims to accelerate discovery of how genes control organ function and disease.
The research team, led by Whitehead Institute Member Jonathan Weissman and then-graduate student in his lab Reuben Saunders, along with Xiaowei Zhuang, the David B. Arnold Professor of Science at Harvard University, and then-postdoc in her lab Will Allen, created a system that can test hundreds of different genetic modifications within a single mouse liver while capturing multiple types of data from the same cells.
“Understanding how our organs work requires looking at many different aspects of cell biology at once,” Saunders says. “With Perturb-Multi, we can see how turning off specific genes changes not just what other genes are active, but also how proteins are distributed within cells, how cellular structures are organized, and where cells are located in the tissue. It’s like having multiple specialized microscopes all focused on the same experiment.”
“This approach accelerates discovery by both allowing us to test the functions of many different genes at once, and then for each gene, allowing us to measure many different functional outputs or cell properties at once—and we do that in intact tissue from animals,” says Zhuang, who is also an HHMI Investigator.
A more efficient approach to genetic studies
Traditional genetic studies in mice often turn off one gene in an animal, and then observe what changes in that gene’s absence to learn about what the gene does. The researchers designed their approach to turn off hundreds of different genes across a single liver, while still only turning off one gene per cell—using what is known as a mosaic approach. This allowed them to study the roles of hundreds of individual genes at once in a single animal. The researchers then collected diverse types of data from cells across the same liver to get a full picture of the consequences of turning off the genes.
“Each cell serves as its own experiment, and because all the cells are in the same animal, we eliminate the variability that comes from comparing different mice,” Saunders says. “Every cell experiences the same physiological conditions, diet, and environment, making our comparisons much more precise.”
“The challenge we faced was that tissues, to perform their functions, rely on thousands of genes, expressed in many different cells, working together. Each gene, in turn, can control many aspects of a cell’s function. Testing these hundreds of genes in mice using current methods would be extremely slow and expensive—near impossible in practice,” Allen says.
Revealing new biology through combined measurements
The team applied Perturb-Multi to study genetic controls of liver physiology and function. Their study led to discoveries in three important aspects of liver biology: fat accumulation in liver cells—a precursor to liver disease; stress responses; and hepatocyte zonation (how liver cells specialize, assuming different traits and functions, based on their location within the liver).
One striking finding emerged from studying genes that, when disrupted, cause fat accumulation in liver cells. The imaging data revealed that four different genes all led to similar fat droplet accumulation, but the sequencing data showed they did so through three completely different mechanisms.
“Without combining imaging and sequencing, we would have missed this complexity entirely,” Saunders says. “The imaging told us which genes affect fat accumulation, while the sequencing revealed whether this was due to increased fat production, cellular stress, or other pathways. This kind of mechanistic insight could be crucial for developing targeted therapies for fatty liver disease.”
The researchers also discovered new regulators of liver cell zonation. Unexpectedly, the newly discovered regulators include genes involved in modifying the extracellular matrix—the scaffolding between cells. “We found that cells can change their specialized functions without physically moving to a different zone,” Saunders says. “This suggests that liver cell identity is more flexible than previously thought.”
Technical innovation enables new science
Developing Perturb-Multi required solving several technical challenges. The team created new methods for preserving the content of interest in cells—RNA and proteins—during tissue processing, for collecting many types of imaging data and single-cell gene expression data from tissue samples that have been fixed with a preservative, and for integrating multiple types of data from the same cells.
“Overcoming the inherent complexity of biology in living animals required developing new tools that bridge multiple disciplines – including, in this case, genomics, imaging, and AI,” Allen says.
The two components of Perturb-Multi—the imaging and sequencing assays—together, applied to the same tissue, provide insights that are unattainable through either assay alone.
“Each component had to work perfectly while not interfering with the others,” says Weissman, who is also a professor of biology at the Massachusetts Institute of Technology and an HHMI Investigator. “The technical development took considerable effort, but the payoff is a system that can reveal biology we simply couldn’t see before.”
Expanding to new organs and other contexts
The researchers plan to expand Perturb-Multi to other organs, including the brain, and to study how genetic changes affect organ function under different conditions like disease states or dietary changes.
“We’re also excited about using the data we generate to train machine learning models,” adds Saunders. “With enough examples of how genetic changes affect cells, we could eventually predict the effects of mutations without having to test them experimentally—a ‘virtual cell’ that could accelerate both research and drug development.”
“Perturbation data are critical for training such AI models and the paucity of existing perturbation data represents a major hindrance in such ‘virtual cell’ efforts,” Zhuang says. “We hope Perturb-Multi will fill this gap by accelerating the collection of perturbation data.”
The approach is designed to be scalable, with the potential for genome-wide studies that test thousands of genes simultaneously. As sequencing and imaging technologies continue to improve, the researchers anticipate that Perturb-Multi will become even more powerful and accessible to the broader research community.
“Our goal is to keep scaling up. We plan to do genome-wide perturbations, study different physiological conditions, and look at different organs,” says Weissman. “That we can now collect so many types of data from so many cells, at speed, is going to be critical for building AI models like virtual cells, and I think it’s going to help us answer previously unsolvable questions about health and disease.”
Reuben A. Saunders, William E. Allen, Xingjie Pan, Jaspreet Sandhu, Jiaqi Lu, Thomas K. Lau, Karina Smolyar, Zuri A. Sullivan, Catherine Dulac, Jonathan S. Weissman, Xiaowei Zhuang. “Perturb-Multimodal: a Platform for Pooled Genetic Screens with Sequencing and Imaging in Intact Mammalian Tissue.” Cell, June 12, 2025. DOI: 10.1016/j.cell.2025.05.022.