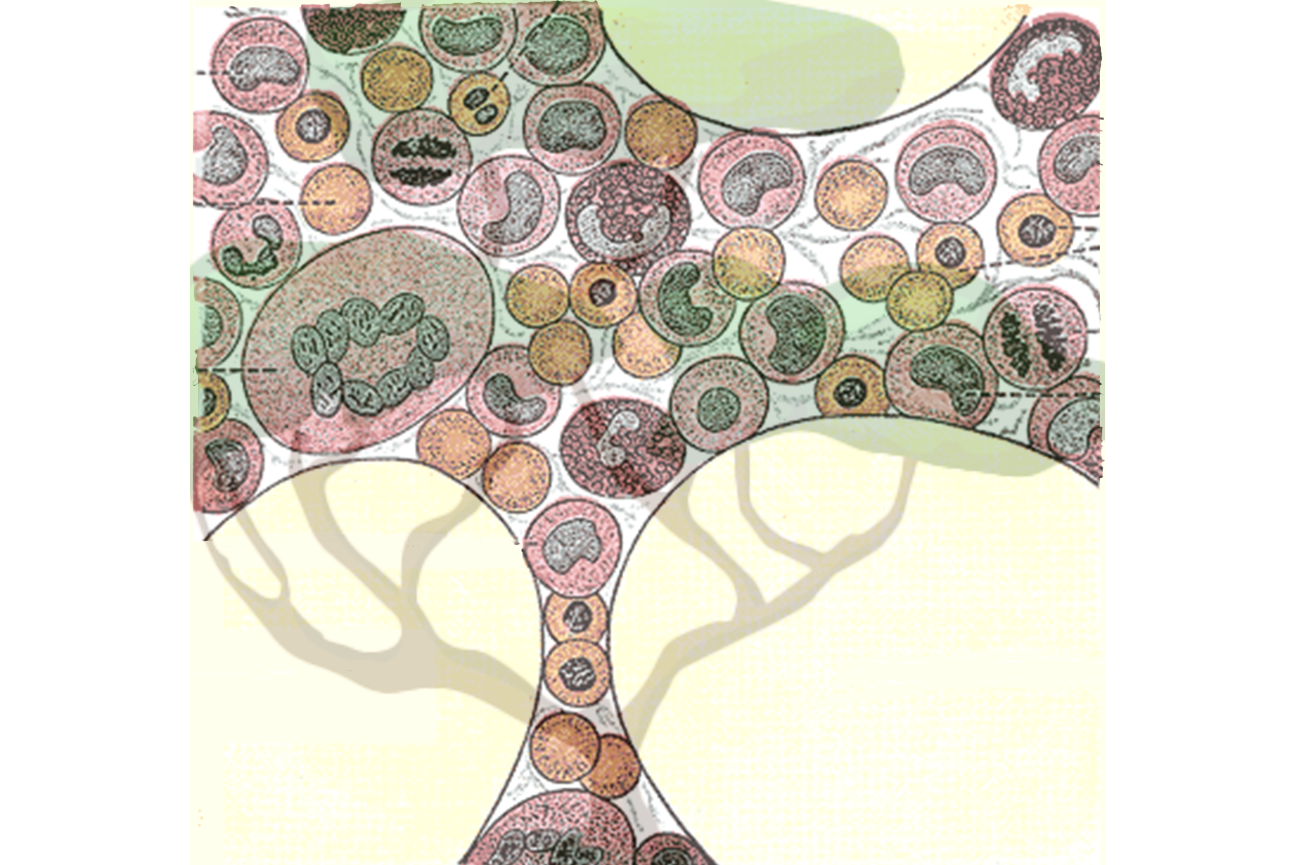
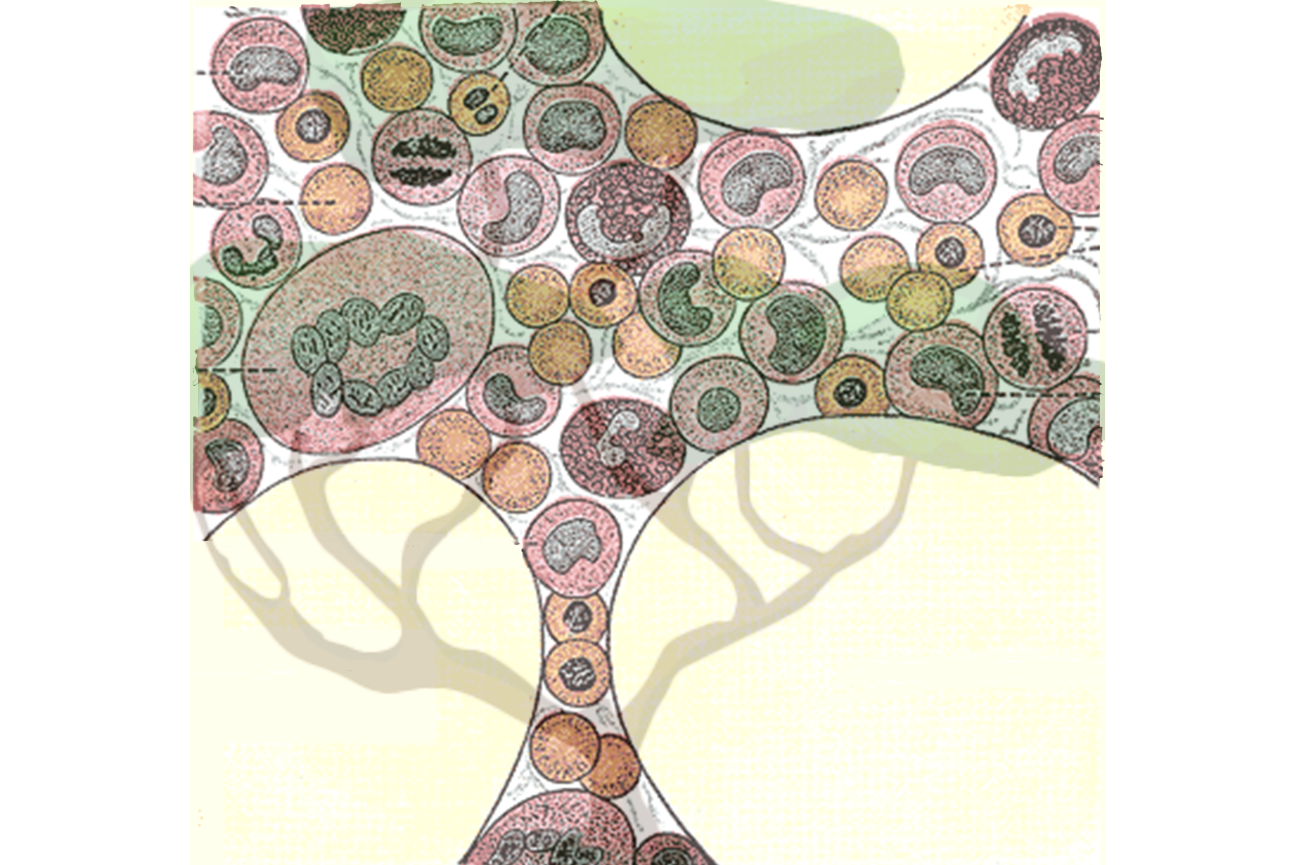
Sergey Ovchinnikov uses phylogenetic inference, protein structure prediction/determination, protein design, deep learning, energy-based models, and differentiable programming to tackle evolutionary questions at environmental, organismal, genomic, structural, and molecular scales, with the aim of developing a unified model of protein evolution.
Humans split away from our closest animal relatives, chimpanzees, and formed our own branch on the evolutionary tree about seven million years ago. In the time since—brief, from an evolutionary perspective—our ancestors evolved the traits that make us human, including a much bigger brain than chimpanzees and bodies that are better suited to walking on two feet. These physical differences are underpinned by subtle changes at the level of our DNA. However, it can be hard to tell which of the many small genetic differences between us and chimps have been significant to our evolution.
New research from Whitehead Institute Member Jonathan Weissman; University of California, San Francisco Assistant Professor Alex Pollen; Weissman lab postdoc Richard She; Pollen lab graduate student Tyler Fair; and colleagues uses cutting edge tools developed in the Weissman lab to narrow in on the key differences in how humans and chimps rely on certain genes. Their findings, published in the journal Cell on June 20, may provide unique clues into how humans and chimps have evolved, including how humans became able to grow comparatively large brains.
Studying function rather than genetic code
Only a handful of genes are fundamentally different between humans and chimps; the rest of the two species’ genes are typically nearly identical. Differences between the species often come down to when and how cells use those nearly identical genes. However, only some of the many differences in gene use between the two species underlie big changes in physical traits. The researchers developed an approach to narrow in on these impactful differences.
Their approach, using stem cells derived from human and chimp skin samples, relies on a tool called CRISPR interference (CRISPRi) that Weissman’s lab developed. CRISPRi uses a modified version of the CRISPR/Cas9 gene editing system to effectively turn off individual genes. The researchers used CRISPRi to turn off each gene one at a time in a group of human stem cells and a group of chimp stem cells. Then they looked to see whether or not the cells multiplied at their normal rate. If the cells stopped multiplying as quickly or stopped altogether, then the gene that had been turned off was considered essential: a gene that the cells need to be active–producing a protein product–in order to thrive. The researchers looked for instances in which a gene was essential in one species but not the other as a way of exploring if and how there were fundamental differences in the basic ways that human and chimp cells function.
By looking for differences in how cells function with particular genes disabled, rather than looking at differences in the DNA sequence or expression of genes, the approach ignores differences that do not appear to impact cells. If a difference in gene use between species has a large, measurable effect at the level of the cell, this likely reflects a meaningful difference between the species at a larger physical scale, and so the genes identified in this way are likely to be relevant to the distinguishing features that have emerged over human and chimp evolution.
“The problem with looking at expression changes or changes in DNA sequences is that there are many of them and their functional importance is unclear,” says Weissman, who is also a professor of biology at the Massachusetts Institute of Technology and an Investigator with the Howard Hughes Medical Institute. “This approach looks at changes in how genes interact to perform key biological processes, and what we see by doing that is that, even on the short timescale of human evolution, there has been fundamental rewiring of cells.”
After the CRISPRi experiments were completed, She compiled a list of the genes that appeared to be essential in one species but not the other. Then he looked for patterns. Many of the 75 genes identified by the experiments clustered together in the same pathways, meaning the clusters were involved in the same biological processes. This is what the researchers hoped to see. Individual small changes in gene use may not have much of an effect, but when those changes accumulate in the same biological pathway or process, collectively they can cause a substantive change in the species. When the researchers’ approach identified genes that cluster in the same processes, this suggested to them that their approach had worked and that the genes were likely involved in human and chimp evolution.
“Isolating the genetic changes that made us human has been compared to searching for needles in a haystack because there are millions of genetic differences, and most are likely to have negligible effects on traits,” Pollen says. “However, we know that there are lots of small effect mutations that in aggregate may account for many species differences. This new approach allows us to study these aggregate effects, enabling us to weigh the impact of the haystack on cellular functions.”
Researchers think bigger brains may rely on genes regulating how quickly cells divide
One cluster on the list stood out to the researchers: a group of genes essential to chimps, but not to humans, that help to control the cell cycle, which regulates when and how cells decide to divide. Cell cycle regulation has long been hypothesized to play a role in the evolution of humans’ large brains. The hypothesis goes like this: Neural progenitors are the cells that will become neurons and other brain cells. Before becoming mature brain cells, neural progenitors divide multiple times to make more of themselves. The more divisions that the neural progenitors undergo, the more cells the brain will ultimately contain—and so, the bigger it will be. Researchers think that something changed during human evolution to allow neural progenitors to spend less time in a non-dividing phase of the cell cycle and transition more quickly towards division. This simple difference would lead to additional divisions, each of which could essentially double the final number of brain cells.
Consistent with the popular hypothesis that human neural progenitors may undergo more divisions, resulting in a larger brain, the researchers found that several genes that help cells to transition more quickly through the cell cycle are essential in chimp neural progenitor cells but not in human cells. When chimp neural progenitor cells lose these genes, they linger in a non-dividing phase, but when human cells lose them, they keep cycling and dividing. These findings suggest that human neural progenitors may be better able to withstand stresses—such as the loss of cell cycle genes—that would limit the number of divisions the cells undergo, enabling humans to produce enough cells to build a larger brain.
“This hypothesis has been around for a long time, and I think our study is among the first to show that there is in fact a species difference in how the cell cycle is regulated in neural progenitors,” She says. “We had no idea going in which genes our approach would highlight, and it was really exciting when we saw that one of our strongest findings matched and expanded on this existing hypothesis.”
More subjects lead to more robust results
Research comparing chimps to humans often uses samples from only one or two individuals from each species, but this study used samples from six humans and six chimps. By making sure that the patterns they observed were consistent across multiple individuals of each species, the researchers could avoid mistaking the naturally occurring genetic variation between individuals as representative of the whole species. This allowed them to be confident that the differences they identified were truly differences between species.
The researchers also compared their findings for chimps and humans to orangutans, which split from the other species earlier in our shared evolutionary history. This allowed them to figure out where on the evolutionary tree a change in gene use most likely occurred. If a gene is essential in both chimps and orangutans, then it was likely essential in the shared ancestor of all three species; it’s more likely for a particular difference to have evolved once, in a common ancestor, than to have evolved independently multiple times. If the same gene is no longer essential in humans, then its role most likely shifted after humans split from chimps. Using this system, the researchers showed that the changes in cell cycle regulation occurred during human evolution, consistent with the proposal that they contributed to the expansion of the brain in humans.
The researchers hope that their work not only improves our understanding of human and chimp evolution, but also demonstrates the strength of the CRISPRi approach for studying human evolution and other areas of human biology. Researchers in the Weissman and Pollen labs are now using the approach to better understand human diseases—looking for the subtle differences in gene use that may underlie important traits such as whether someone is at risk of developing a disease, or how they will respond to a medication. The researchers anticipate that their approach will enable them to sort through many small genetic differences between people to narrow in on impactful ones underlying traits in health and disease, just as the approach enabled them to narrow in on the evolutionary changes that helped make us human.
Two faculty members from the MIT Department of Biology have been selected by the Howard Hughes Medical Institute (HHMI) for the inaugural cohort of HHMI Freeman Hrabowski Scholars.
Seychelle Vos, the Robert A. Swanson Career Development Professor of Life Sciences, and Hernandez Moura Silva, an assistant professor of biology and core member of the Ragon Institute of MGH, MIT and Harvard, are among 31 early-career faculty selected for their potential to become leaders in their research fields and to create diverse and inclusive lab environments in which everyone can thrive, according to a press release.
Freeman Hrabowski Scholars are appointed to a five-year term, renewable for a second five-year term after a successful progress evaluation. Each scholar will receive up to $8.6 million over 10 years, including full salary, benefits, a research budget, and scientific equipment. In addition, they will participate in professional development to advance their leadership and mentorship skills.
The Freeman Hrabowski Scholars Program represents a key component of HHMI’s diversity, equity, and inclusion goals. Over the next 20 years, HHMI expects to hire and support up to 150 Freeman Hrabowski Scholars — appointing roughly 30 scholars every other year for the next 10 years. The institute has committed up to $1.5 billion for the Freeman Hrabowski Scholars to be selected over the next decade. The program was named for Freeman A. Hrabowski III, president emeritus of the University of Maryland at Baltimore County, who played a major role in increasing the number of scientists, engineers, and physicians from backgrounds underrepresented in science in the United States.
Seychelle Vos
Seychelle Vos studies how DNA organization impacts gene expression at the atomic level, using cryogenic electron microscopy (cryo-EM), X-ray crystallography, biochemistry, and genetics. Human cells contain about 2 meters of DNA, which is packed so tightly that its entirety is contained within the nucleus, which is only a few microns across. Although DNA needs to be compacted, it also needs to be accessible to, and readable by, the cell’s molecular machinery.
Vos received a BS in genetics from the University of Georgia in 2008 and a PhD from University of California at Berkeley in 2013. During her postdoctoral research at the Max Planck Institute for Biophysical Chemistry in Germany, she determined how the molecular machine responsible for gene expression is regulated near gene promoters.
Vos joined MIT as an assistant professor of biology in fall 2019.
“I am very humbled and honored to have been named a HHMI Freeman Hrabowski Scholar,” Vos says. “It would not have been possible without the hard work of my lab and the help of my colleagues. It provides us with the support to achieve our ambitious research goals.”
Hernandez Moura Silva
Hernandez Moura Silva studies the role of immune cells in the maintenance and normal function of our bodies and tissues, beyond their role in battling infection. Specifically, he looks at a specific type of immune cell called a macrophage and its role in the proper function of white adipose tissue — our fat. White adipose tissue in a healthy state is highly populated by macrophages, including very abundant ones known as “vasculature-associated adipose tissue macrophages,” which are located around the blood vessels. When the activity of these adipose macrophages is disrupted, there are changes in the proper function of the white adipose tissue, which may ultimately link to disease. By understanding macrophage function in healthy tissues, Hernandez hopes to learn how to restore tissue homeostasis in disease.
Hernandez Moura Silva received a BS in biology in 2005 and an MSc in molecular biology in 2008 from the University of Brazil. He received his PhD in 2011 from the University of São Paulo Heart Institute. Silva pursued his postdoctoral work as the Bernard Levine Postdoctoral Fellow in immunology and immuno-metabolism at the New York University School of Medicine Skirball Institute of Biomolecular Medicine.
He joined MIT as an assistant professor of biology in 2022. He is also a core member of the Ragon Institute.
“For an immigrant coming from an underrepresented group, it’s a huge privilege to be granted this opportunity from HHMI that will empower me and my lab to shape the next generation of scientists and provide an environment where people can feel welcome and encouraged to do the science that they love and be successful,” Silva says. “It also aligns with MIT’s commitment to increase diversity and opportunity across the Institute and to become a place where all people can thrive.”
Billions of times a day, every day of our lives, cells receive signals to initiate the process of cell death. This strategic cell death, also called apoptosis, is one of the tools multicellular organisms use to maintain tissues and regulate immune responses: damaged, old, or superfluous cells are given the green light to, as it were, turn out the lights for the last time.
Programmed cell death is both extremely powerful and extremely regulated: for example, the careful culling of cells between our digits during embryonic development reveals fingers and toes. When programmed cell death goes awry, however, it can have serious consequences. Cells left unchecked can divide unstoppably and aggressively, leading to cancer. Dysregulated apoptotic pathways have also been implicated in neurodegenerative diseases like Alzheimer’s, where unrestrained cell death may play a part in the severity of the disease.
MIT Professor H. Robert Horvitz ‘68 shared a Nobel prize in 2002 for his foundational research on the genetics of programmed cell death and organ development in the nematode, a microscopic roundworm. Horvitz discovered that ced-9, a key gene in programmed cell death in nematodes, was similar in structure and function to the human gene bcl-2.
Targeting members of the BCL-2 protein family has already shown promise in the fight against cancer. For example, approved by the FDA in 2016, the oral drug Venetoclax is a BCL-2 inhibitor used to treat certain types of leukemia.
In a study published online Jan. 26 in Structure, Fiona Aguilar PhD ‘22 (Keating lab) and collaborators focused on a member of the BCL-2 protein family called BAK. When it is active, BAK promotes mitochondrial outer membrane disruption, leading to cell death, and is therefore referred to as a pro-apoptotic protein. But precisely how BAK becomes activated – or inhibited – is unknown.
“A greater understanding of BAK activation is interesting both from a fundamental biochemical and biophysical perspective as well as from the more translational one of BAK as a potential therapeutic target,” says lead author Fiona Aguilar.
BAK exists in two different forms: an inactive monomer and an active oligomer. A few activators of BAK (BIM, truncated BID, and PUMA) have already been identified and these proteins bind directly to BAK, leading to the model that binding of activators trigger changes in protein shape that allow BAK to transition from the inactive to active forms. To further explore this idea, Aguilar identified and characterized a number of other peptides that bind to and regulate BAK. To identify new peptide binders, the team used cell-surface display screening and computational protein design methods, including techniques developed by Keating lab alum Gevorg Grigoryan– dTERMen and TERMify – that use protein structural data to generate new protein sequences likely to bind a protein of interest.
In total, Aguilar et al. discovered 10 diverse new peptide binders of BAK that regulate its function.
Interestingly, some of the BAK-binding peptides inhibited activation rather than promoting it. Aguilar et al. found that inhibitors and activators of BAK shared many characteristics including structure as well as binding affinity and kinetics – the strength and rate that binders associate with and dissociate from BAK.
Newly identified activators had sequences both dissimilar from one another and from the previously known BAK activators BIM, truncated BID, and PUMA. The similarity of the sequence was not necessarily a good indicator of activation or inhibition. For example, an inhibitor and an activator differed by just two amino acids.
Aguilar and colleagues solved the crystal structures of two inhibitor-BAK complexes and one activator-BAK complex and found that the activator interacted with BAK with similar geometry as the two inhibitors. Also, the two inhibitors have only about 40% sequence identity, but bind very similarly to BAK.
Amy Keating, the senior author on the study, says “Fiona was tireless in identifying new peptides, testing their interactions with BAK, determining their functions, and solving structures to look for differences between activators and inhibitors. We were surprised that peptides with such different behaviors shared such common interaction properties.”
Although the puzzle is not yet solved, Aguilar believes the “transition state” between inactive and active forms of BAK is key.
“We think of activators as peptides that preferentially bind to the BAK transition state, whereas inhibitors are those that preferentially bind to the monomeric state,” Aguilar says. “Overall, we should be thinking more about the transition state, what steps are necessary to reach the transition state, and how to target the transition state.”
This study also added two sequences in the human proteome – BNIP5 and PXT1 – to the repertoire of known BAK binders. Not much is known about these sequences, Aguilar says, but the fact that they activate BAK could indicate that they may play a role in apoptotic pathways that have not yet been determined.
“The finding is something that people in the field are pretty excited about,” Aguilar says.
Ultimately, work remains to establish what characteristics of the binders determine their function, and how binding to BAK triggers the conformational changes that activate or inhibit this complex protein.
“It’s still unclear what it is about these sequences that trigger the allosteric network leading to BAK activation, but at least for now we can rule out the hypothesis that binding mode, affinity, and kinetics fully determine how this occurs,” Aguilar says.
Aguilar suggests that it will be interesting also to explore how these peptides interact with BAX, another pro-apoptotic protein in the BCL-2 family that is both structurally and functionally similar to BAK.
Fiona Aguilar is lead author and Amy Keating is senior author; Bob Grant and graduate students Sebastian Swanson, Dia Ghose, and Bonnie Su contributed. Collaborators Stacey Yu and Kristopher Sarosiek, from the Harvard T.H. Chan School of Public Health, helped with cell-based experiments. The research was funded by a National Institute of General Medical Sciences award, the MIT School of Science Fellowship in Cancer Research award, the John W. Jarve (1978) Seed Fund for Science Innovation (MIT) award, an award from the National Cancer Institute, a National Institute of Diabetes and Digestive and Kidney Diseases award, and Alex’s Lemonade Stand Foundation for Childhood Cancers award.
About 70 percent of all human proteins include at least one sequence consisting of a single amino acid repeated many times, with a few other amino acids sprinkled in. These “low-complexity regions” are also found in most other organisms.
The proteins that contain these sequences have many different functions, but MIT biologists have now come up with a way to identify and study them as a unified group. Their technique allows them to analyze similarities and differences between LCRs from different species, and helps them to determine the functions of these sequences and the proteins in which they are found.
Using their technique, the researchers have analyzed all of the proteins found in eight different species, from bacteria to humans. They found that while LCRs can vary between proteins and species, they often share a similar role — helping the protein in which they’re found to join a larger-scale assembly such as the nucleolus, an organelle found in nearly all human cells.
“Instead of looking at specific LCRs and their functions, which might seem separate because they’re involved in different processes, our broader approach allows us to see similarities between their properties, suggesting that maybe the functions of LCRs aren’t so disparate after all,” says Byron Lee, an MIT graduate student.
The researchers also found some differences between LCRs of different species and showed that these species-specific LCR sequences correspond to species-specific functions, such as forming plant cell walls.
Lee and graduate student Nima Jaberi-Lashkari are the lead authors of the study, which appears today in eLife. Eliezer Calo, an assistant professor of biology at MIT, is the senior author of the paper.
Large-scale study
Previous research has revealed that LCRs are involved in a variety of cellular processes, including cell adhesion and DNA binding. These LCRs are often rich in a single amino acid such as alanine, lysine, or glutamic acid.
Finding these sequences and then studying their functions individually is a time-consuming process, so the MIT team decided to use bioinformatics — an approach that uses computational methods to analyze large sets of biological data — to evaluate them as a larger group.
“What we wanted to do is take a step back and instead of looking at individual LCRs, to try to take a look at all of them and to see if we could observe some patterns on a larger scale that might help us figure out what the ones that have assigned functions are doing, and also help us learn a bit about what the ones that don’t have assigned functions are doing,” Jaberi-Lashkari says.
To do that, the researchers used a technique called dotplot matrix, which is a way to visually represent amino acid sequences, to generate images of each protein under study. They then used computational image processing methods to compare thousands of these matrices at the same time.
Using this technique, the researchers were able to categorize LCRs based on which amino acids were most frequently repeated in the LCR. They also grouped LCR-containing proteins by the number of copies of each LCR type found in the protein. Analyzing these traits helped the researchers to learn more about the functions of these LCRs.
As one demonstration, the researchers picked out a human protein, known as RPA43, that has three lysine-rich LCRs. This protein is one of many subunits that make up an enzyme called RNA polymerase 1, which synthesizes ribosomal RNA. The researchers found that the copy number of lysine-rich LCRs is important for helping the protein integrate into the nucleolus, the organelle responsible for synthesizing ribosomes.
Biological assemblies
In a comparison of the proteins found in eight different species, the researchers found that some LCR types are highly conserved between species, meaning that the sequences have changed very little over evolutionary timescales. These sequences tend to be found in proteins and cell structures that are also highly conserved, such as the nucleolus.
“These sequences seem to be important for the assembly of certain parts of the nucleolus,” Lee says. “Some of the principles that are known to be important for higher order assembly seem to be at play because the copy number, which might control how many interactions a protein can make, is important for the protein to integrate into that compartment.”
The researchers also found differences between LCRs seen in two different types of proteins that are involved in nucleolus assembly. They discovered that a nucleolar protein known as TCOF contains many glutamine-rich LCRs that can help scaffold the formation of assemblies, while nucleolar proteins with only a few of these glutamic acid-rich LCRs could be recruited as clients (proteins that interact with the scaffold).
Another structure that appears to have many conserved LCRs is the nuclear speckle, which is found inside the cell nucleus. The researchers also found many similarities between LCRs that are involved in forming larger-scale assemblies such as the extracellular matrix, a network of molecules that provides structural support to cells in plants and animals.
The research team also found examples of structures with LCRs that seem to have diverged between species. For example, plants have distinctive LCR sequences in the proteins that they use to scaffold their cell walls, and these LCRs are not seen in other types of organisms.
The researchers now plan to expand their LCR analysis to additional species.
“There’s so much to explore, because we can expand this map to essentially any species,” Lee says. “That gives us the opportunity and the framework to identify new biological assemblies.”
The research was funded by the National Institute of General Medical Sciences, National Cancer Institute, the Ludwig Center at MIT, a National Institutes of Health Pre-Doctoral Training Grant, and the Pew Charitable Trusts.
We use the tiny, transparent jellyfish, Clytia hemisphaerica, to ask questions at the interface of nervous system evolution, development, regeneration, and function. Our foundation is in systems neuroscience, where we use genetic and optical techniques to examine how behavior arises from the activity of networks of neurons. Building from this work, we investigate how the Clytia nervous system is so robust, both to the constant integration of newborn neurons and following large-scale injury. Lastly, we use Clytia’s evolutionary position to study principles of nervous system evolution and make inferences about the ultimate origins of nervous systems.