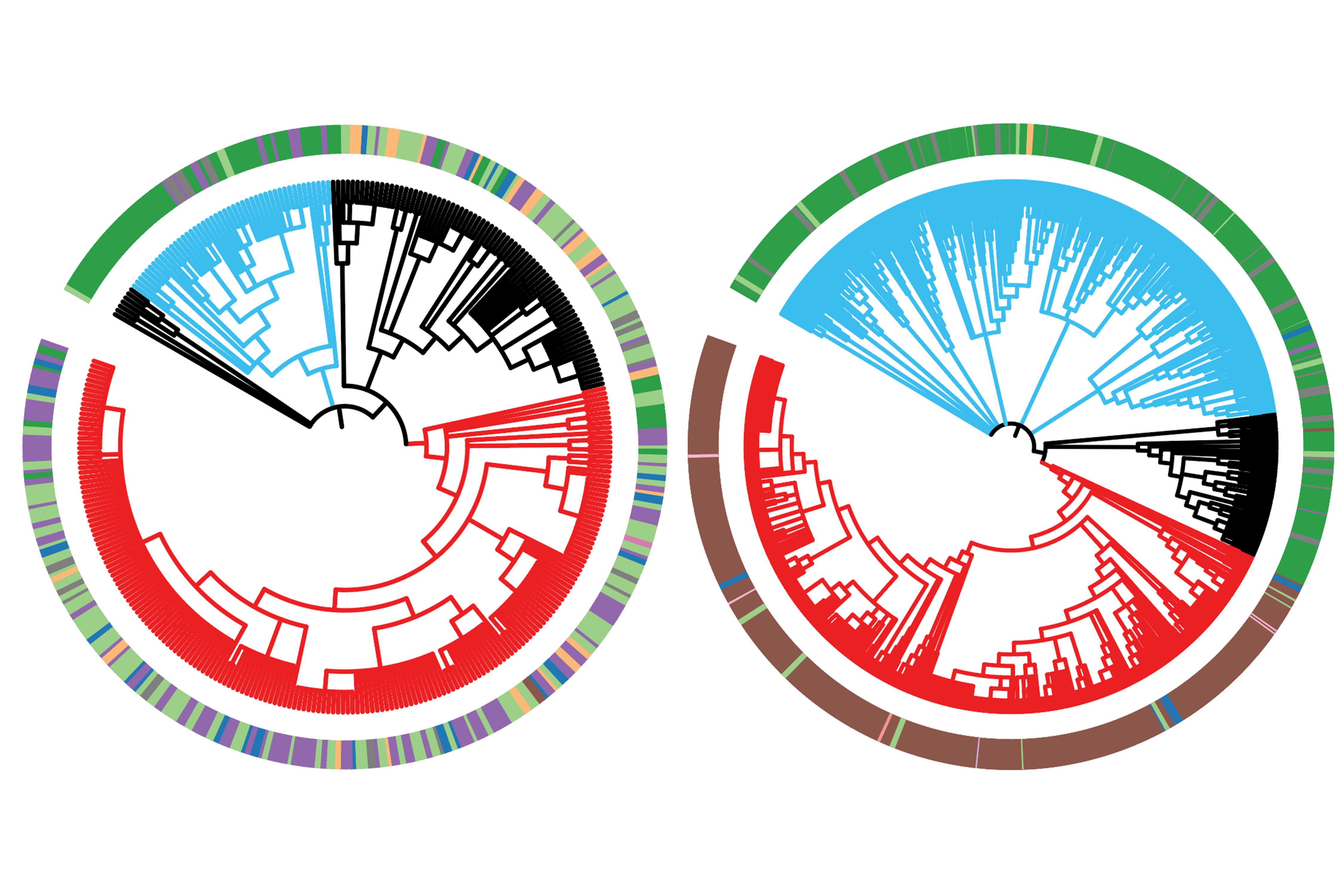
Family trees of lung cancer cells reveal how cancer evolves from its earliest stages to an aggressive form capable of spreading throughout the body.
Greta Friar | Whitehead Institute
May 5, 2022
Over time, cancer cells can evolve to become resistant to treatment, more aggressive, and metastatic — capable of spreading to additional sites in the body and forming new tumors. The more of these traits that a cancer evolves, the more deadly it becomes. Researchers want to understand how cancers evolve these traits in order to prevent and treat deadly cancers, but by the time cancer is discovered in a patient, it has typically existed for years or even decades. The key evolutionary moments have come and gone unobserved.
MIT Professor Jonathan Weissman and collaborators have developed an approach to track cancer cells through the generations, allowing researchers to follow their evolutionary history. This lineage-tracing approach uses CRISPR technology to embed each cell with an inheritable and evolvable DNA barcode. Each time a cell divides, its barcode gets slightly modified. When the researchers eventually harvest the descendants of the original cells, they can compare the cells’ barcodes to reconstruct a family tree of every individual cell, just like an evolutionary tree of related species. Then researchers can use the cells’ relationships to reconstruct how and when the cells evolved important traits. Researchers have used similar approaches to follow the evolution of the virus that causes Covid-19, in order to track the origins of variants of concern.
Weissman and collaborators have used their lineage-tracing approach before to study how metastatic cancer spreads throughout the body. In their latest work, Weissman; Tyler Jacks, the Daniel K. Ludwig Scholar and David H. Koch Professor of Biology at MIT; and computer scientist Nir Yosef, associate professor at the University of California at Berkeley and the Weizmann Institute of Science, record their most comprehensive cancer cell history to date. The research, published today in Cell, tracks lung cancer cells from the very first activation of cancer-causing mutations. This detailed tumor history reveals new insights into how lung cancer progresses and metastasizes, demonstrating the wealth of understanding that lineage tracing can provide.
“This is a new way of looking at cancer evolution with much higher resolution,” says Weissman, who is a professor of biology at MIT, a member of the Whitehead Institute for Biomedical Research, and an investigator with Howard Hughes Medical Institute. “Previously, the critical events that cause a tumor to become life-threatening have been opaque because they are lost in a tumor’s distant past, but this gives us a window into that history.”
In order to track cancer from its very beginning, the researchers developed an approach to simultaneously trigger cancer-causing mutations in cells and start recording the cells’ history. They engineered mice such that when their lung cells were exposed to a tailor-made virus, that exposure activated a cancer-causing mutation in the Kras gene and deactivated tumor suppressing gene Trp53 in the cells, as well as activating the lineage tracing technology. The mouse model, developed in Jacks’ lab, was also engineered so that lung cancer would develop in it very similarly to how it would in humans.
“In this model, cancer cells develop from normal cells and tumor progression occurs over an extended time in its native environment. This closely replicates what occurs in patients,” Jacks says. Indeed, the researchers’ findings closely align with data about disease progression in lung cancer patients.
The researchers let the cancer cells evolve for several months before harvesting them. They then used a computational approach developed in their previous work to reconstruct the cells’ family trees from their modified DNA barcodes. They also measured gene expression in the cells using RNA sequencing to characterize each individual cell’s state. With this information, they began to piece together how this type of lung cancer becomes aggressive and metastatic.
“Revealing the relationships between cells in a tumor is key to making sense of their gene expression profiles and gaining insight into the emergence of aggressive states,” says Yosef, who is a co-corresponding author on both the current work and the previous lineage tracing paper.
The results showed significant diversity between subpopulations of cells within the same tumor. In this model, cancer cells evolved primarily through inheritable changes to their gene expression, rather than through genetic mutations. Certain subpopulations had evolved to become more fit — better at growth and survival — and more aggressive, and over time they dominated the tumor. Genes that the researchers identified as commonly expressed in the fittest cells could be good candidates for possible therapeutic targets in future research. The researchers also discovered that metastases originated only from these groups of dominant cells, and only late in their evolution. This is different from what has been proposed for some other cancers, in which cells may gain the ability to metastasize early in their evolution. This insight could be important for cancer treatment; metastasis is often when cancers become deadly, and if researchers know which types of cancer develop the ability to metastasize in this stepwise manner, they can design interventions to stop the progression.
“In order to develop better therapies, it’s important to understand the fundamental principles that tumors adopt to develop,” says co-first author Dian Yang, a Damon Runyon Postdoctoral Fellow in Weissman’s lab. “In the future, we want to be able to look at the state of the cancer cells when a patient comes in, and be able to predict how that cancer’s going to evolve, what the risks are, and what is the best treatment to stop that evolution.”
The researchers also figured out important details of the evolutionary paths that cancer subpopulations take to become fit and aggressive. Cells evolve through different states, defined by key characteristics that the cell has at that point in time. In this cancer model the researchers found that early on, cells in a tumor quickly diversified, switching between many different states. However, once a subpopulation landed in a particularly fit and aggressive state, it stayed there, dominating the tumor from that stable state. Furthermore, the ultimately dominant cells seemed to follow one of two distinct paths through different cell states. Either of those paths could then lead to further progression that enabled cancers to enter aggressive “mesenchymal” cell states, which are linked to metastasis.
After the researchers thoroughly mapped the cancer cells’ evolutionary paths, they wondered how those paths would be affected if the cells experienced additional cancer-linked mutations, so they deactivated one of two additional tumor suppressors. One of these affected which state cells stabilized in, while the other led cells to follow a completely new evolutionary pathway to fitness.
The researchers hope that others will use their approach to study all kinds of questions about cancer evolution, and they already have a number of questions in mind for themselves. One goal is to study the evolution of therapeutic resistance, by seeing how cancers evolve in response to different treatments. Another is to study how cancer cells’ local environments shape their evolution.
“The strength of this approach is that it lets us study the evolution of cancers with fine-grained detail,” says co-first author Matthew Jones, a graduate student in the Weissman and Yosef labs. “Every time there is a shift from bulk to single-cell analysis in a technology or approach, it dramatically widens the scope of the biological insights we can attain, and I think we are seeing something like that here.”