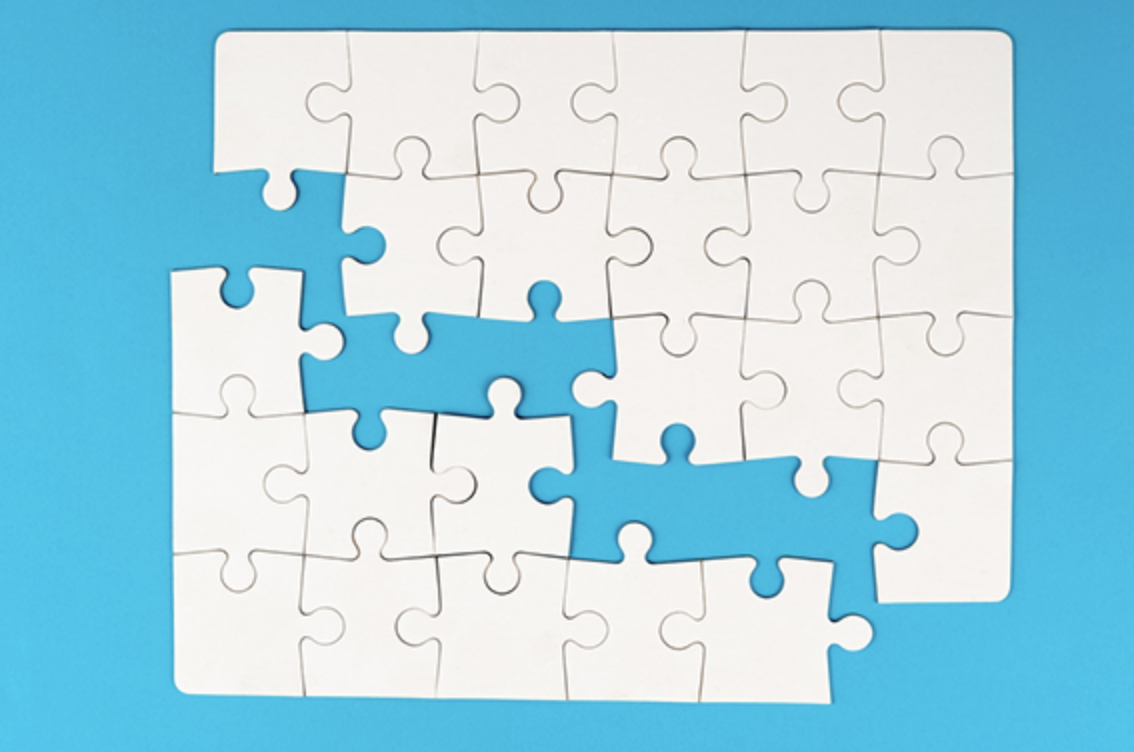
Greta Friar | Whitehead Institute
December 5, 2019
In order for the instructions contained within a gene to ultimately execute some function in the body, the nucleotides, or letters, that make up the gene’s DNA sequence must be “read” and used to produce a messenger RNA (mRNA). This mRNA must then be translated into a functional protein. A number of different pathways within the cell influence this essential biological process, informing whether, when, and to what extent a gene is expressed. A major class of such regulators are microRNAs (miRNAs). These minute RNAs—they are, on average, 22 nucleotides long—join with a protein called Argonaute to cause certain mRNAs to be degraded, which in turn decreases the amount of translation of those mRNAs into their functional protein forms. Scientists have identified hundreds of miRNAs that are common amongst mammals and other vertebrate animals, and most mammalian mRNAs are targeted by at least one of these miRNAs—an indication of their pervasive importance to our biology. Accurately predicting how any particular miRNA will affect gene expression in a cell is important for understanding our own biology, and might facilitate the design of therapeutic drugs that affect or utilize miRNAs, but the complexity of the miRNA pathway makes this sort of prediction difficult.
The success rate with which a miRNA is able to repress a specific gene (by degrading its mRNA) is called its targeting efficacy, and researchers have used a variety of models to calculate it, with mixed results. In the past, researchers have treated miRNAs as a group and looked at average behavior in order to make predictions, because there simply wasn’t enough data specific to individual miRNAs available to do otherwise. However, Whitehead Institute Member David Bartel, who is also a professor of biology at the Massachusetts Institute of Technology and a Howard Hughes Medical Institute investigator, graduate student Sean McGeary, and former graduate student Kathy Lin collected a massive amount of data on six miRNAs, and from that foundation developed an improved predictive model for all individual miRNAs. Their findings, published online in Science on December 5, provide unprecedented accuracy and granularity in miRNA targeting prediction.
“We used to focus our attention on microRNA targeting patterns that were consistent, because that consistency gave us confidence in what we were seeing,” Bartel says, “but with the robust results of this research, we can now pay attention to differences between individual miRNAs.”
Bartel and the Whitehead Institute Bioinformatics and Research Computing group operate one of the go-to resources for prediction of miRNAs’ targets and target efficacy, known as TargetScan. This latest research will be used to update TargetScan, giving scientists around the world an even more useful reference tool for research involving miRNA-mediated regulation of gene expression.
To understand miRNA targeting, researchers need to identify the particular sites within an mRNA sequence where the miRNA can bind, and they additionally need to know how strong the interaction will be at each site—the binding affinity. In general, a miRNA will bind to an mRNA when there is a match between at least six of the first eight nucleotides of the miRNA and a complementary sequence of nucleotides somewhere on the mRNA. The two sequences are like rows of puzzle pieces being pushed together: if each puzzle piece slots into the corresponding piece, the rows combine into one locked puzzle—the miRNA binds its target. If the pieces don’t fit together, the rows can’t connect. These sorts of binding sites, perfect matches within the first eight nucleotides of the miRNA, are called canonical site types, and researchers used to think that there was a clear hierarchy between them, with each individual site type conferring a similar amount of repression regardless of the miRNA identity. But that’s not what McGeary observed.
McGeary looked at six miRNAs and developed a method to measure, for each miRNA, relative binding affinities to a massive collection of RNA sequences.
“I performed experiments that provide vast numbers of measurements, which collectively inform us on how well a miRNA will bind to an mRNA,” McGeary says.
These measurements, as well as further calculations that McGeary made from them, formed a novel, rich pool of data with which to improve miRNA targeting prediction. From their experiments, the researchers found that the expected targeting hierarchy of canonical sites did not apply to all miRNAs. An individual miRNA might actually have a stronger affinity to one of the canonical sites lower in the expected hierarchy than another. Furthermore, the group discovered that the miRNAs each had unique noncanonical binding sites, some of which were sites that contained at least one mismatch but were still able to bind miRNA. The researchers found many instances in which a miRNA bound more strongly to one of its noncanonical sites than to some of its canonical sites, despite the imperfect or unusual pairing of the noncanonical sites.
“As humans, we like to classify things into discrete buckets with discrete characteristics,” Lin says. “But to build a model that is quantitative, you have to recognize that each miRNA and target interaction is different.”
Factors in a target site’s environment contribute to the individuality of target interactions, as they can affect the structural accessibility of the site for binding. In particular, the researchers found that the four nucleotides closest to a target site could have a huge, even 100-fold combined impact on affinity.
With their high-resolution data, the researchers were able to rigorously verify a supposition within the miRNA research community: that the strength with which a miRNA binds to a target site is the major determinant for how effective that miRNA will be at degrading that mRNA. This striking correlation between site affinity and targeting efficacy also allowed them to create a biochemical model of miRNA targeting that used the vast collection of affinity measurements to predict the efficacy of repression of every mRNA in cell, significantly out-performing all existing models of miRNA targeting. They then used machine learning, in the form of a convolutional neural network developed by Lin, to extend the improved predictions to all miRNAs without the need to generate additional data.
Altogether, these findings paint a much richer picture of miRNA-mediated gene repression. The new level of specificity in miRNA targeting prediction will provide all researchers working on the subject with better information about the impact of a given miRNA in a cell.
This work was supported by the NIH and Howard Hughes Medical Institute.
Written by Greta Friar
***
David Bartel’s primary affiliation is with Whitehead Institute for Biomedical Research, where his laboratory is located and all his research is conducted. He is also a professor of biology at Massachusetts Institute of Technology and investigator with the Howard Hughes Medical Institute.
***
Citation:
“The biochemical basis of microRNA targeting efficacy”
Science, online December 5, 2019, DOI: 10.1126/science.aav1741
Sean E. McGeary (1,2,3†), Kathy S. Lin (1,2,3,4†), Charlie Y. Shi (1,2,3), Thy Pham (1,2,3), Namita Bisaria (1,2,3), Gina M. Kelley (1,2,3), and David P. Bartel (1,2,3,4)
- Howard Hughes Medical Institute, Cambridge, MA, 02142, USA
- Whitehead Institute for Biomedical Research, Cambridge, MA, 02142, USA
- Department of Biology, Massachusetts Institute of Technology, Cambridge, MA 02139, USA
- Computational and Systems Biology Program, Massachusetts Institute of Technology, Cambridge, MA, 02139, USA
†These authors contributed equally to this work.